Why accuracy matters more than precision
October 20, 2021 •Kevin Davis
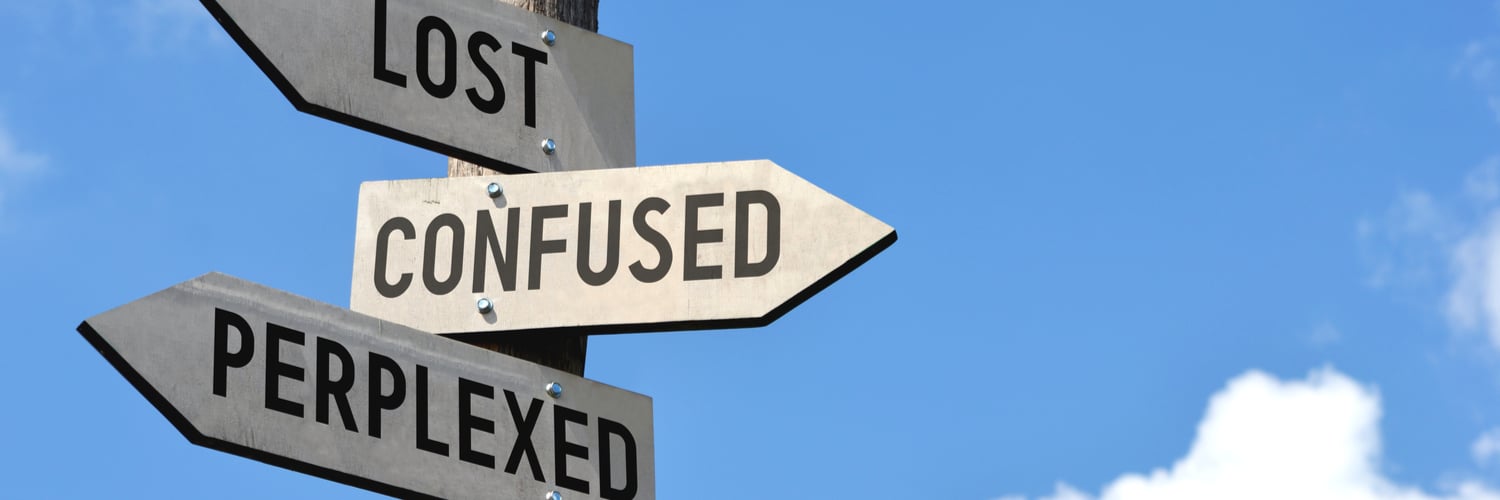
Here’s a question for your analytics team or agency: which is better, precision or accuracy?
If your team is good, they’ll say both. If they’re really good, they’ll say accuracy. Why? And why does it matter? Don’t they both boil down to the same thing?
Not really. Just ask Peter Crouch (more on that later).
There's a huge difference between precision and accuracy. You can be precise but not accurate but you can never be accurate if you’re not precise. And if you’re on the Board of a D2C retailer or ecommerce business, you need to understand the difference.
In the language of data and forecasting, definitions matter and any misalignment at Board level can have serious trickle-down consequences. Brands are awash with data and bombarded with a never-ending stream of gadgets and gizmos that promise to make more precise forecasting based on ever-more granular segmentation and analysis. But all this comes with a serious health warning attached.
The danger with forecasting is that you risk being too precise and therefore less accurate. And any loss in accuracy means money wasted and revenue not generated. Nobody wants that.
But why does accuracy matter more? Here’s a quick (jargon-free) explainer.
This is what accurate versus precise forecasting looks like in practice:
Here, the centre of the bullseye is your future performance. A forecast that focusses heavily on precision will generate results that are very exact and precise… but may be wildly inaccurate. Focus on accuracy, however, and we know that we’ll end up the right ballpark, even if we’re not right on the bullseye (though we’re usually very, very close).
When we’re forecasting, we use a set of assumptions that we know to be true to predict the future performance of your business. These predictions can only be as good as the assumptions we use. Think of exit polls in a general election: the bigger the data sample, the closer the results will be to the true election outcome. The same thing happens in your business. When your segments are too precise and small, the margin of error in your forecasting gets bigger and the model becomes less accurate. The challenge is to find the right level of precision for each circumstance – that will be different for every business.
Precision bias is a tempting (and dangerous) pitfall. We are more likely to believe a number to be accurate if it is precise because, we feel, someone has done a lot of clever sums in the background to come up with this one very precise figure. This is not the case but it does explain why so many businesses sacrifice accuracy and focus on precision instead.
We recently met with a D2C business who had broken their forecast down in minute detail by revenue-driver and were trying to make usable assumptions based on each individual driver. In other words, they were looking at all their revenue drivers one by one and trying to extrapolate business-wide insight based on tiny microsegments of (incorrect) data.
They were focussed on precision instead of accuracy and it was a mess. Any small errors across the data set snowballed into an aggregate that produced a terrible forecast that was completely inaccurate and unusable. At best, it was a waste of time. At worst, it could have derailed their stock-buying, revenue predictions, PE negotiations, operations and warehouse capacity, returns… you name it.
Take another example (and this is where Crouchy comes in). We could tell you that Peter Crouch is roughly 6’5’’. Or we could say he’s exactly 173.5cm. One statement is fairly accurate (more or less) and the other is precise (but completely wrong). Neither is 100% correct but, if you’re trying to buy him a pair of jeans for Christmas, you’re better off going with the accurate assumption, not the precise one.
Used correctly, precision forecasting is a very useful tool. But if you buy an off-the-shelf product that’s focussed on precision and isn’t tailored to your business, you’re buying yourself a world of pain.
The simple things matter most: the size of your database, your re-order rate, your ambitions and your historical sales stability will all have a big impact on what your model needs to look like. Logically, your forecasting methods need to be tailored to meet the specific needs of your business. If you’re thinking about buying one-size-fits-all or less refined models, be sure that they’re right for your business and will give accurate results: don’t get swayed by the precision or granularity of a forecast as it won’t guarantee a more accurate result.
When it comes down to it, if you’re not a data whizz, you don’t need to know exactly how the forecasting works. All you need to know is that your teams (be they in-house or at an agency) are using the right assumptions so that the results are accurate and can be trusted. You need proof.
Many direct-to-consumer brands struggle to get a clear and accurate forecast because simply looking at last year’s performance and expecting to do the same thing again isn’t enough anymore (for one thing, it leads to inaccurate stock buying, increased discounting and reduced profits). When 25 companies represent 90% of the retail industry’s increase in global market capitalization since March 2020, it’s time to look at where those 25 are investing their time and energy. Unsurprisingly, those top 25 are highly data-optimised. As always, it pays to learn what the best of the best are doing – and making it work for your business.
Good forecasting unlocks growth in your business because it shows you how much you can afford to invest and how your business should perform over time. Do it correctly and it will make your business sing. Do it incorrectly and you’ll either throttle growth (by underinvesting) or stopper cashflow (by overinvesting).
It’s never a bad time to talk about forecasting and it’s always worth questioning the forecasts you’re working from. After all, they form the basis for all your Board decisions so they’re worth getting right.
At more2, we work with over 100 brands, helping them to build accurate, trustworthy forecasts that are fit for purpose and will help them make good decisions based on their customer behaviour and specific unit economics. If you’d like to learn more about how we do it (and whether we can help your brand to understand your data better), get in touch below!
Kevin Davis
Kevin is more2's Chief Data Scientist, working with our clients’ customer data to help answer the questions that really matter. Kevin joined more2 in 2018 after spending 18 years working both client- and agency-side, specialising in using customer data to solve problems and drive strategic action. At more2, Kevin and his team of analysts, data scientists and business intelligence specialists deliver over 1,000 bespoke analytical projects a year to our clients.
Catherine Stoney
Cat is our Analytics Manager and the heart of our Analytics team. She's been with us since 2014 and has worked on nearly all of our 100+ clients, leading the team with her strategic outlook and experience and delivering world-class data and insight to her clients.
Want to chat about anything you've read here? Drop us a line here and one of the team will be in touch very soon!